When I first started automating AI-generated images for news stories, the goal was straightforward: take the latest headlines, generate a visually compelling representation using DALL·E 3, and ensure that the images matched both the tone and context of the news. The concept was solid, but as with any evolving project, refinements were necessary to make the process more efficient, reliable, and creatively engaging.
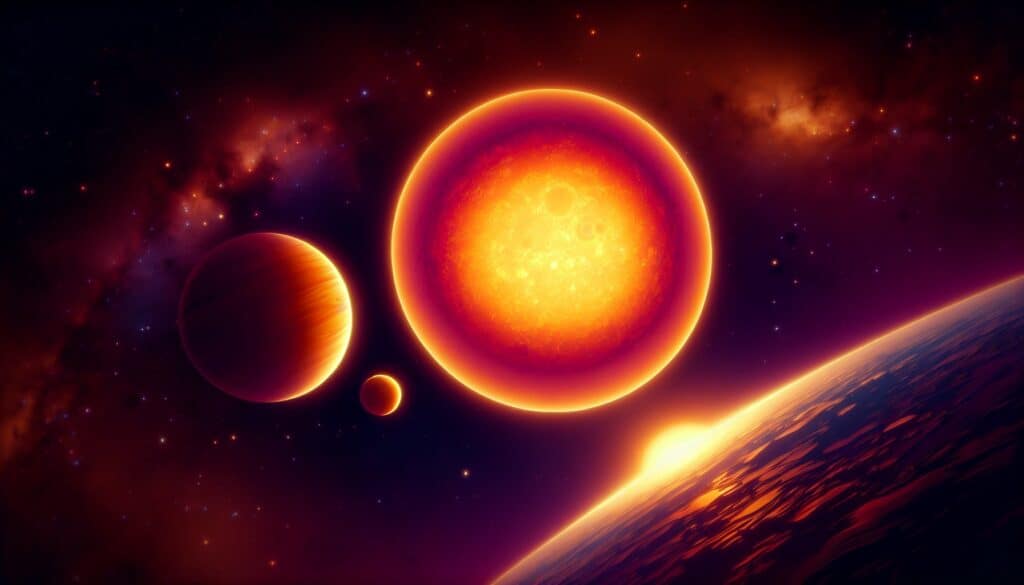
The Initial Concept
At first, the workflow was simple:
- Retrieve a set of major news headlines.
- Generate a DALL·E-friendly prompt summarizing the news.
- Send the prompt to DALL·E and retrieve an image.
- Save the image for use in news articles.
This worked, but I quickly realized that improvements were needed to refine the accuracy and efficiency of the process.
Key Enhancements and Iterations
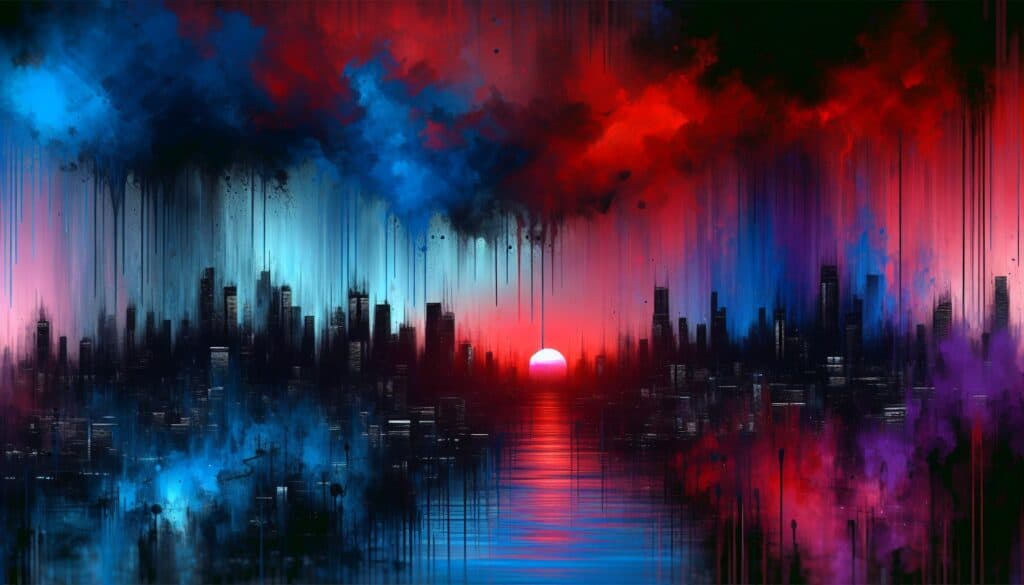
Through multiple iterations, the process evolved into something much more streamlined and flexible:
1. Improving Prompt Engineering
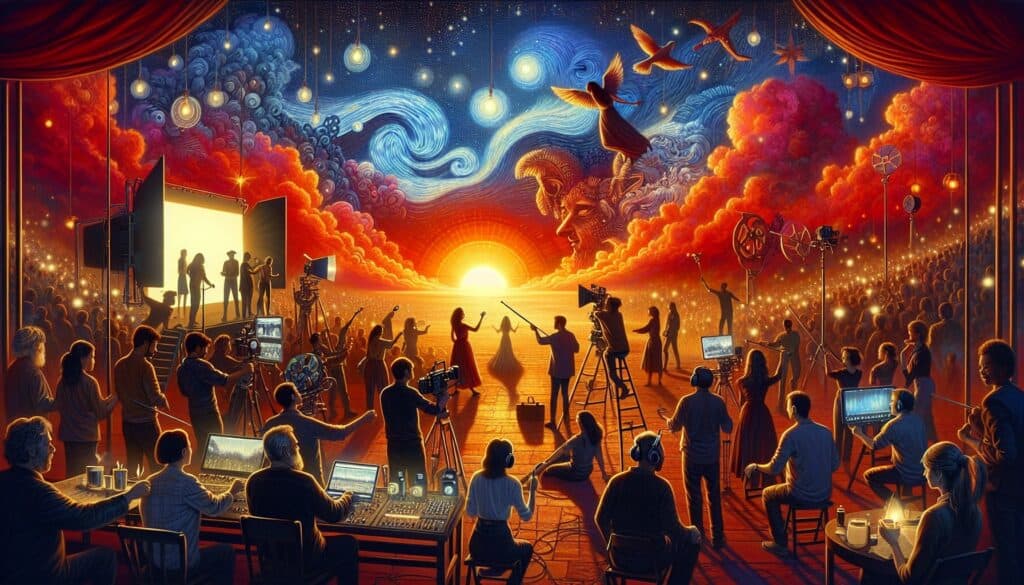
Originally, prompts were generated with minimal structuring, which led to inconsistent results. To address this, I implemented a structured prompt-building process that:
- Ensures each image aligns with the news theme.
- Balances descriptive storytelling with AI interpretability.
- Optimizes word choice to avoid misinterpretation by DALL·E.
2. Handling API Calls More Efficiently
Initially, each step required a separate request, which was inefficient. By modularizing API calls, I made the system:
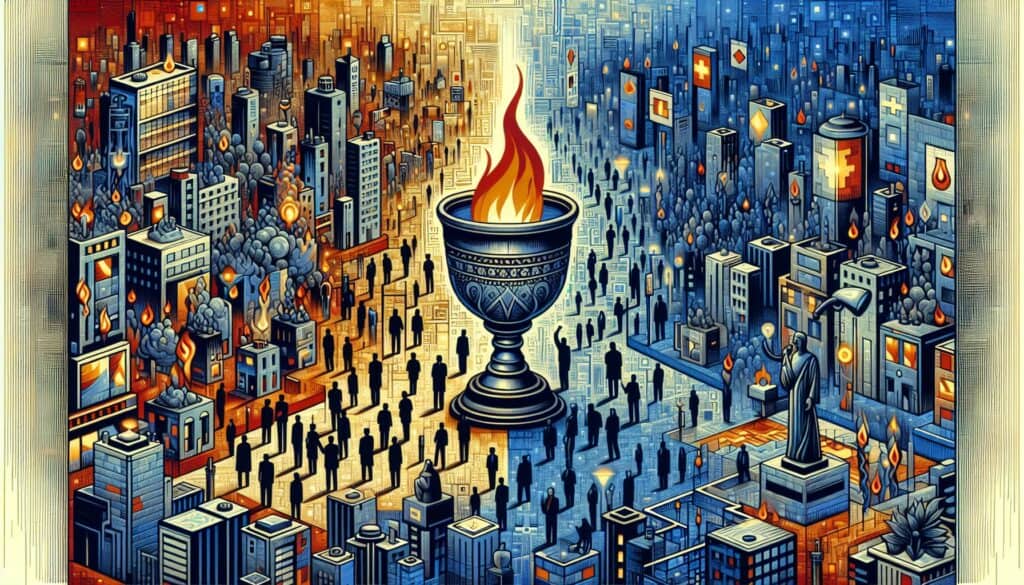
- Easier to debug and maintain.
- More adaptable to future updates.
- Capable of handling large batches of requests without bottlenecks.
3. Refining Image Curation
Instead of generating a single image per prompt, I added:
- Multiple Style Variants – Each image is now created in different artistic styles, allowing for greater editorial flexibility.
- Automated Quality Filtering – The system now detects problematic generations and flags them for review, reducing manual oversight.
4. Automating Image Retrieval and Storage
Initially, I had to manually extract and download images from JSON responses. The updated workflow now:
- Automatically downloads images upon generation.
- Stores metadata alongside each image for easier retrieval and categorization.
5. Enhanced Error Handling and Logging
Occasional errors like rate limits and API hiccups required a more robust handling system. Now, the script:
- Detects and retries failed requests intelligently.
- Logs key API responses for easier debugging and optimization.
- Differentiates between recoverable and critical failures, preventing unnecessary delays.
Lessons Learned
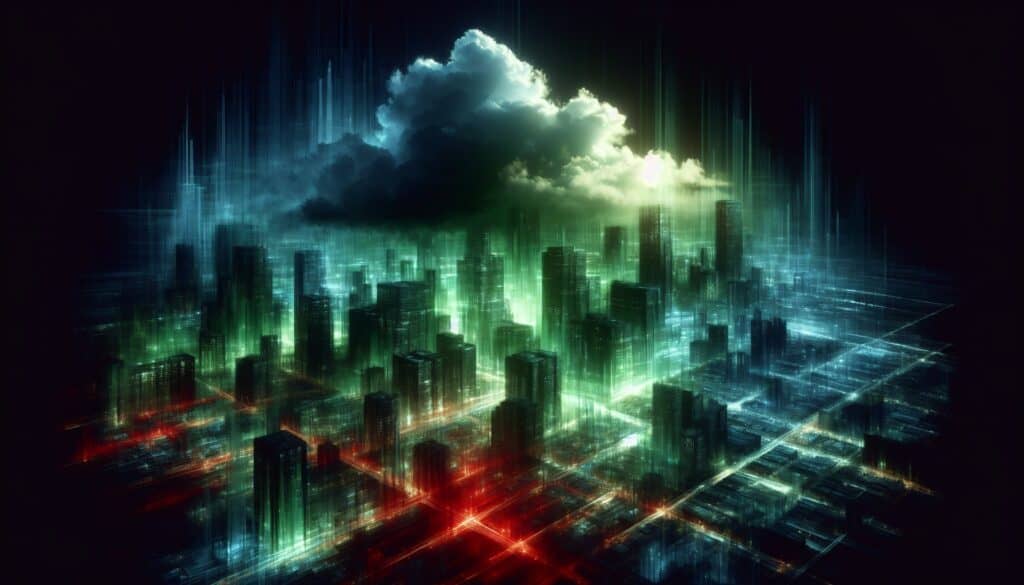
Through these refinements, a few critical lessons emerged:
- Reusable Code Saves Time. Breaking the process into functions rather than hardcoded steps significantly improved flexibility and maintainability.
- AI Prompting Is a Skill. Small variations in wording can lead to dramatically different outputs. Structuring prompts with intent ensures consistency.
- Iteration Drives Improvement. The first working version is rarely the best. Continuous tweaks and refinements yield better results over time.
The Final Workflow (For Now!)
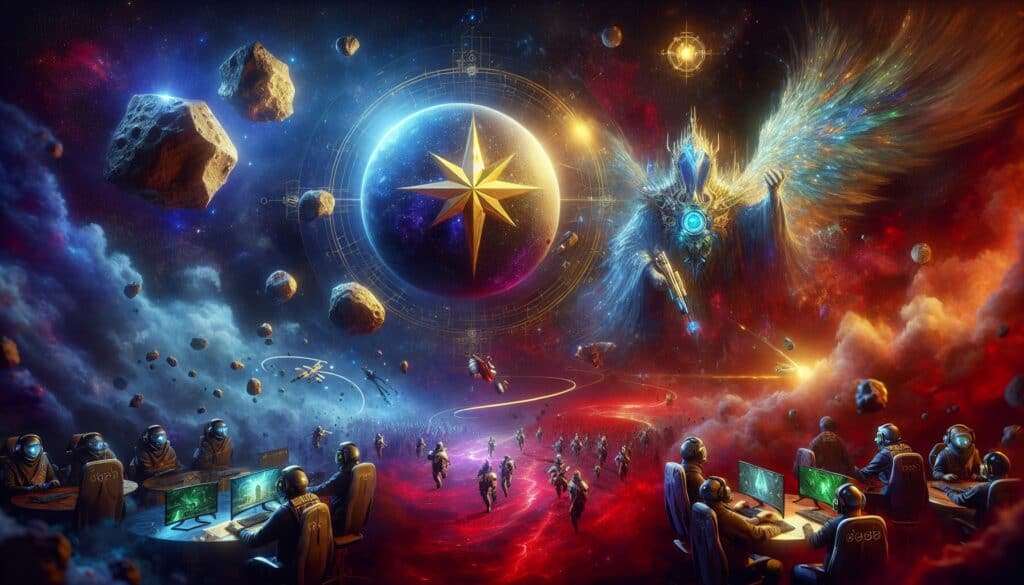
The system now seamlessly:
- ✅ Retrieves and processes the latest news headlines.
- ✅ Generates structured prompts tailored to each news story.
- ✅ Selects a visually distinct, relevant artistic style.
- ✅ Creates multiple image variants for editorial selection.
- ✅ Automatically downloads and organizes images.
This latest iteration is a solid foundation, but I’m always looking ahead. Future improvements could include real-time image curation, integration with other AI tools, or even dynamic animation generation.
What’s next? Maybe AI-driven image captioning? Maybe a real-time news visualization dashboard? The possibilities are endless, and that’s what makes this process so exciting.